The tendency to commit fraud is very much part of every business and capital construction projects are no different. Actually, there are more chances to commit fraud on a project than operational frauds where usually corporate policies and procedures are well enforced and internal or external audit is an ongoing requirement.
When it comes to delivering capital construction projects, there are many actions and transactions that could be labeled as “Project Fraud”. Examples of those actions include:
- Unsubstantiated project decisions
- Under-reported estimates of project cost
- Over-reported estimates of project benefits
- Misuse of requests for Information to cover design errors and omissions
- Improper replacement of specified material and equipment
- Improper inspection and acceptance of work in place
- Unbalanced bids, Over-reported schedule performance to hide project delays
- Wrong forecasting for the cost to complete the remaining project scope
- Unsubstantiated change orders
- Over-estimated change orders
- Over-reported quality progress
- Acceptance of lower-quality deliverables that cannot be used as originally thought
- Delayed and/or improper approvals
- Conflict of interest and kickbacks
- Negligence in protecting client’s interest
- Non-compliance with the contract’s terms and conditions
- Creating unnecessary threats by failing to comply with best practices
- Failing to report threats before they harm the project
- Failing to report opportunities that could have benefited the project
- Lost or misplaced project documents
- Appointing unqualified project team members
- Intended wrong actions or decisions
Detecting a fraud transaction requires having access to all transactions that have the risk of fraud actions as well as the content of the transaction in a format that can recognize its content so it can be used. For example, the bid proposals analysis report helps in identifying six possible fraud transactions. Those transactions include:
- was unqualified bidder invited to bid or not
- was the number of bidders who have submitted bids more than the required minimum
- was any of the submitted bid proposals unbalanced
- was the approved cost estimate in line with the received bid proposals
- was the technical assessment completed
- was the bid proposal analysis reviewed and approved by the authorized individuals as per the Delegation of Authority (DoA) matrix
This means that to be able to detect fraud transactions, for each project-related business process, there should be a predefined template to capture the content of each transaction. For example, for the bid analysis, the template will be used to capture the required data of bidders invited to bid, approved project cost estimate, bid proposal price of each bidder, technical evaluation scoring, and the details of all those individuals who had a role in reviewing and approving the bid proposal analysis report.
Using a Project Management Information System (PMIS) solution like PMWeb, those requirements can be immediately fulfilled if the business processes that have fraud transactions risk are digitized. This will be achieved by having an input template to capture all data needed to perform the business process that will have a workflow for the tasks needed to complete the transaction while abiding with the approval authority levels defined in the Delegation of Authority (DoA) matrix. PMWeb comes ready with the input templates that are common to business processes needed to manage capital construction projects. In addition, PMWeb custom form builder allows creating other input templates that could be needed to manage projects. Regardless of whether it was a default or custom-created template, access rights to each template and fields within a template can be restricted to only those authorized to be part of the business process.
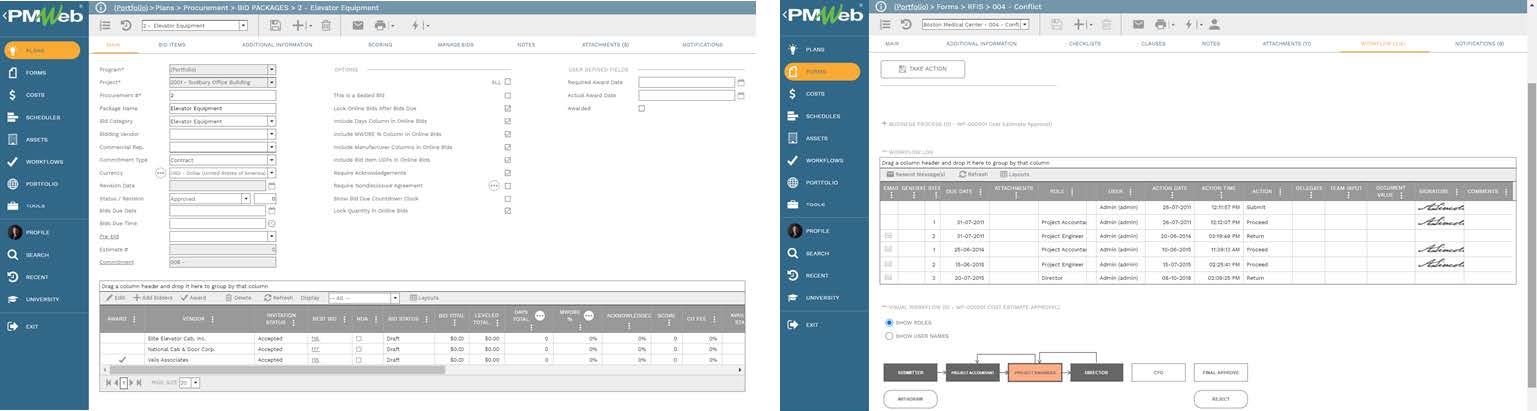
For each transaction of each business process, documents that are relevant to the transaction can be also attached as well as links to other related transactions can be added. Those documents are usually uploaded and stored in the PMWeb document management repository in their specified folder or subfolder to ensure that they are available and accessible whenever a fraud transaction is being investigated.
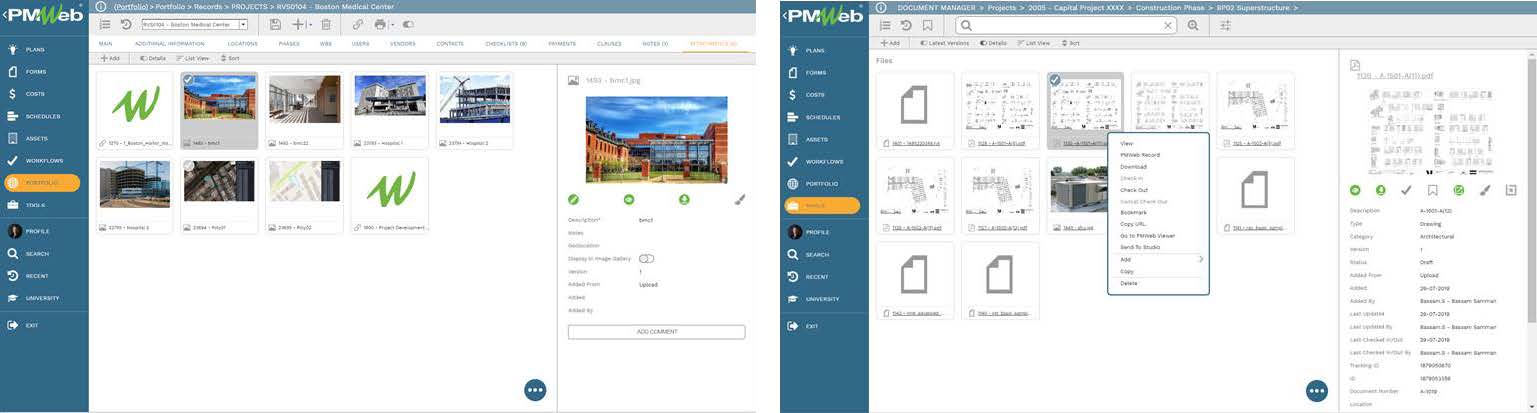
An example of transaction fraud, on a major capital construction project, a contracting company was specifically created to bid on the project. The company used another contracting company registration credentials to be able to bid on the project. This newly formed contracting company had inside information that there were mistakes in the pile design for which there will be a need to redesign and extend the length of piles to what will be stated in the tender documents.
Knowing this information, the contractor unbalanced the bid proposal to show a very low unit price for the pile caps as those will not be subject to change while overpricing the piles unit price for which it was based on the pile length. This enabled the contractor to submit the most competitive offer and for which he was awarded the contract.
After the contract was awarded to this new company and as a result of the post-award soil investigation report, the wrong pile design was identified. This required redesigning the piles for which the pile length was needed to be extended to rectify the mistake. Not only the contractor ended getting a change order with a massive profit margin but also claimed for time extension and other costs associated with the pile redesign effort. Actually, as if those additional costs were not enough, the contractor was paid an extra amount for a directive acceleration to recover those delays.
In other words, at least three major fraud actions were committed by the project owner’s own team that has resulted in major damages and for which the project owner was obliged to cover. In addition to the fraud transaction of having incorrect soil investigation report and wrong design, there were two more fraud transactions, the first was inviting an unqualified contractor to bid while the second was accepting this contractor’s unbalanced bid.Now If the project owner team have had digitized the process for registering and qualifying contractors, those involved in the prequalifying bidder’s business process would have immediately identified that this contracting company lacks the required past experience, financial records, and other documents required for them to be qualified and allowed to bid on the project. The workflow assigned to review and approve the decision to qualify a company will document the actions and comments made by each individual who had a role in the made decision.
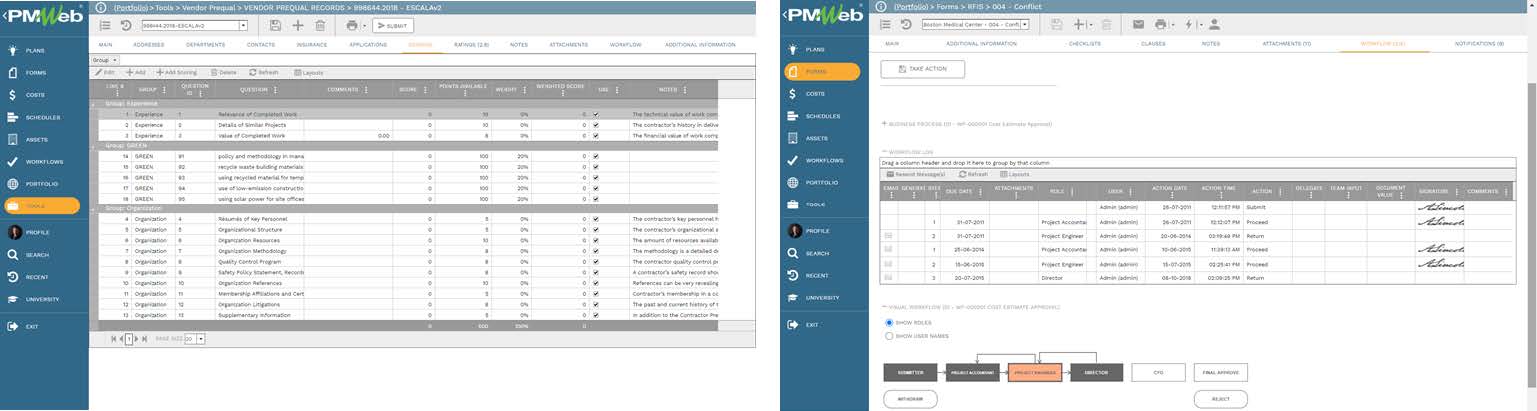
In addition, if the project owner had digitized the bid analysis business process where the proposals for each bidder will be automatically captured by enabling online bidding so they can be compared with the approved cost estimate, any front loading or unbalanced bid items will be immediately identified. Those unbalanced bid items can be considered as red alerts to revisit the tender documents and identify if those were associated with inside knowledge of possible changes to the project scope. The workflow assigned to review and approve the decision to recommend the bidder to be awarded the contract will document the actions and comments made by each individual who had a role in the made decision.
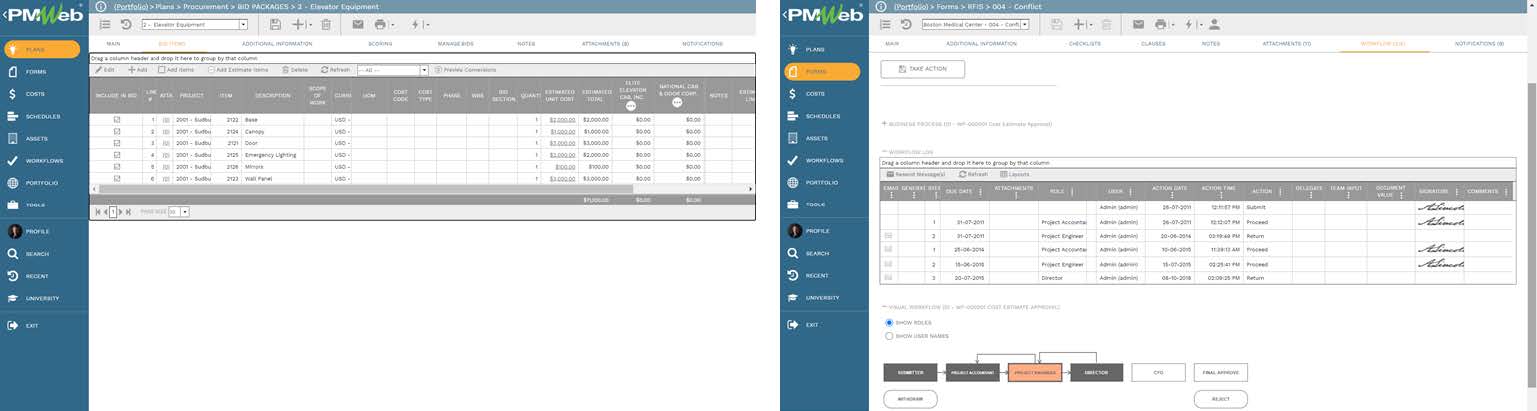
Of course, the captured data for the business processes that are associated with fraud transactions is not always that clear and easy to capture. For example, some design consultants might cause project owners to become liable for the additional costs associated with design errors and omissions. Usually, those errors or discrepancies get identified by the contractor who would raise a Request for Information (RFI) requesting clarifications on the identified ambiguities or errors. The design consultant might recommend issuing a change order for the additional works that will be needed by the contractor to rectify those errors and omissions. Therefore, it is very important that RFIs are associated with the Change Orders that could occur as a result of the answer given to an RFI.
Using the same PMWeb platform, the business processes for Request for Information (RFI) and Change Orders are one of the many default business processes that are readily available in PMWeb. There is even a built-in integration between those two business processes to associate an RFI with a Change Order. This enables tracing the RFI that has caused any Change Order. Of course, the workflow assigned to review and approve the two businesses will document the actions and comments made by each individual who had a role in approving the RFI and Change Order transactions under investigation.For example, the RFI issued by the piling contractor detailing the results of the new soil investigation report and the impact it will have on the existing pile design would have immediately raised a red alert for possible design errors and omissions. In addition, the response made by the design consultant for which it has identified that there could be cost and schedule impact should have again been raised as another red alert.
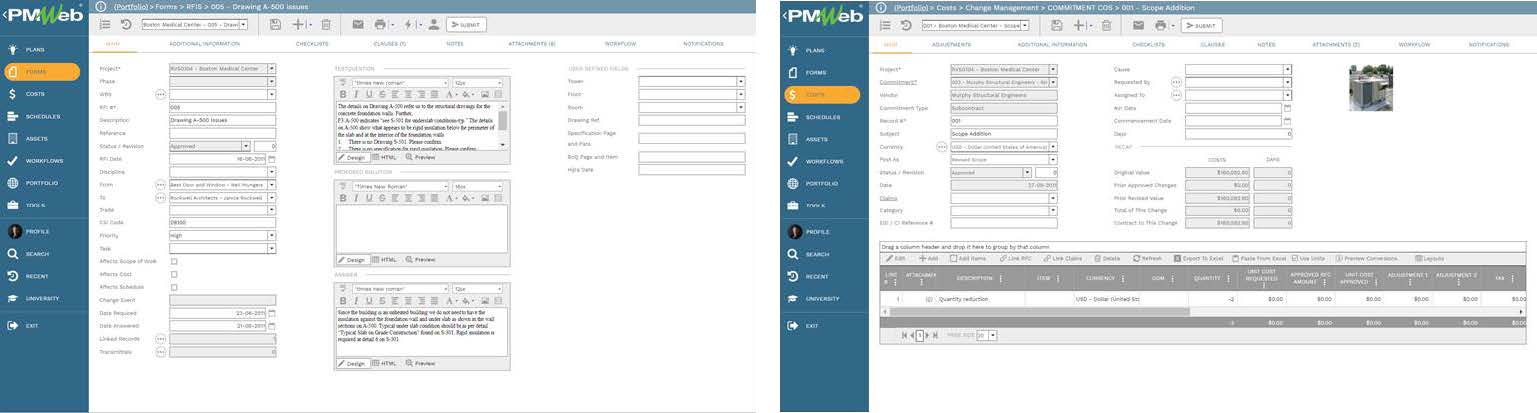
The digitization of the different business processes needed to manage capital construction projects has helped in generating the volume of Big Data that enables taking advantage of machine learning (ML) and artificial intelligence (AI). The use of ML and AI on capital construction projects can improve detecting and flagging fraud actions and transactions. Unlike creating reports to detect fraud transactions based on the queries required to issue alerts if a fraud action has occurred, Fraud Detection with ML and AI helps to have complete data analysis within milliseconds and detects complex patterns in the most efficient way which can be difficult for those reports and individuals to detect.
Machine learning (ML) refers to the analytic techniques that “learn” patterns in datasets without being guided by a human analyst. Artificial intelligence (AI) refers to the broader application of specific kinds of analytics to accomplish tasks like identifying a fraudulent transaction.
Both supervised and unsupervised machine learning models play important roles in fraud detection and must be used. The supervised machine learning model is used to document the previous experience in detecting fraud transactions. The supervised ML model is a model that is trained on a rich set of properly “tagged” transactions. Each transaction is tagged as either fraud or non-fraud. The models are trained by ingesting massive amounts of tagged transaction details in order to learn patterns that best reflect legitimate behaviors. When developing a supervised model, the amount of clean, relevant training data is directly correlated with model accuracy.
This will be possible by digitizing the business processes that are associated with fraudulent transactions. This means that similar to the digitized business processes of contractor prequalification, bid analysis, RFI, and change order detailed above, there is also a need to digitize the many other business processes of the cost estimate, work inspection requests (WIR), material inspection requests (MIR), technical submittals, punch lists, interim payment certificates, claim notices, material alternative submissions, daily reports, baseline schedule review, schedule update review, weekly and monthly progress reports, miscellaneous invoices, non-conformance reports (NCR), risk registers, timesheets, daily reports, staff appraisal among many others. This will enable tagging which of those generated transactions had a fraud action and which did not.
The Unsupervised machine learning models are designed to spot anomalous behavior in cases where tagged transaction data is relatively thin or non-existent. In these cases, a form of self-learning must be employed to surface patterns in the data that are invisible to other forms of analytics. For example, the unsupervised ML model might detect large volumes of RFIs being issued by a contractor as well as a considerable volume of claim notices were also submitted. The unsupervised model might also detect that is a common pattern for all projects that this same contractor has with the project owner.
In addition, the qualification submission for this contractor might show that this contractor has many pending claims with other project owners. Further, the bid comparison report might have also shown that this contractor always bid low to win projects. Those patterns and information of under-bidding, a large volume of RFIs and Claim Notices, and the many projects that have ended with claims and disputes could be an indication that this contractor is a claim-oriented contractor where he depends on claims to gain additional revenue from projects that he wins.
Improving the supervised and unsupervised machine learning models requires digitizing all business processes needed to manage capital construction projects. In addition, it requires that the project owner uses the PMIS platform across their complete projects’ portfolios. There is also the requirement to capture the relevant data for completed projects as this will provide additional data to better train the Machine Learning models.
There are many types of supervised and unsupervised machine learning algorithms. For example, algorithms for the supervised model include linear and logistics regression, random forest, neural network, classification trees, and support vector machines whereas for unsupervised models the algorithms include k-means and Apriori algorithm. Each one of those algorithms has its own advantages and disadvantages and where it is best suited to be used. The data scientists need to be select the algorithms that will achieve the performance requirements for fraud detection.
In conclusion, similar to financial fraud transactions where Machine Learning and Artificial Intelligence have improved the detection of those transactions, ML and AI can improve the detection of fraudulent transactions on capital construction projects. For this to be achievable, the supervised and unsupervised machine learning models need to have access to a large volume of trustworthy data.
Using Project Management Information Systems (PMIS) solutions like PMWeb are crucial for digitizing the hundred-plus business processes needed to deliver capital construction projects. PMWeb will enable organizations to have access to the big data captured from the hundreds of thousands of transactions and associated documents that were carried out on the organization projects’ portfolio. This is the sort of trustworthy big data volume that is needed for Machine Learning (ML) models to learn and better perform. This will enable artificial intelligence (AI) to identify all fraudulent transactions.